The Future of Digital Twins
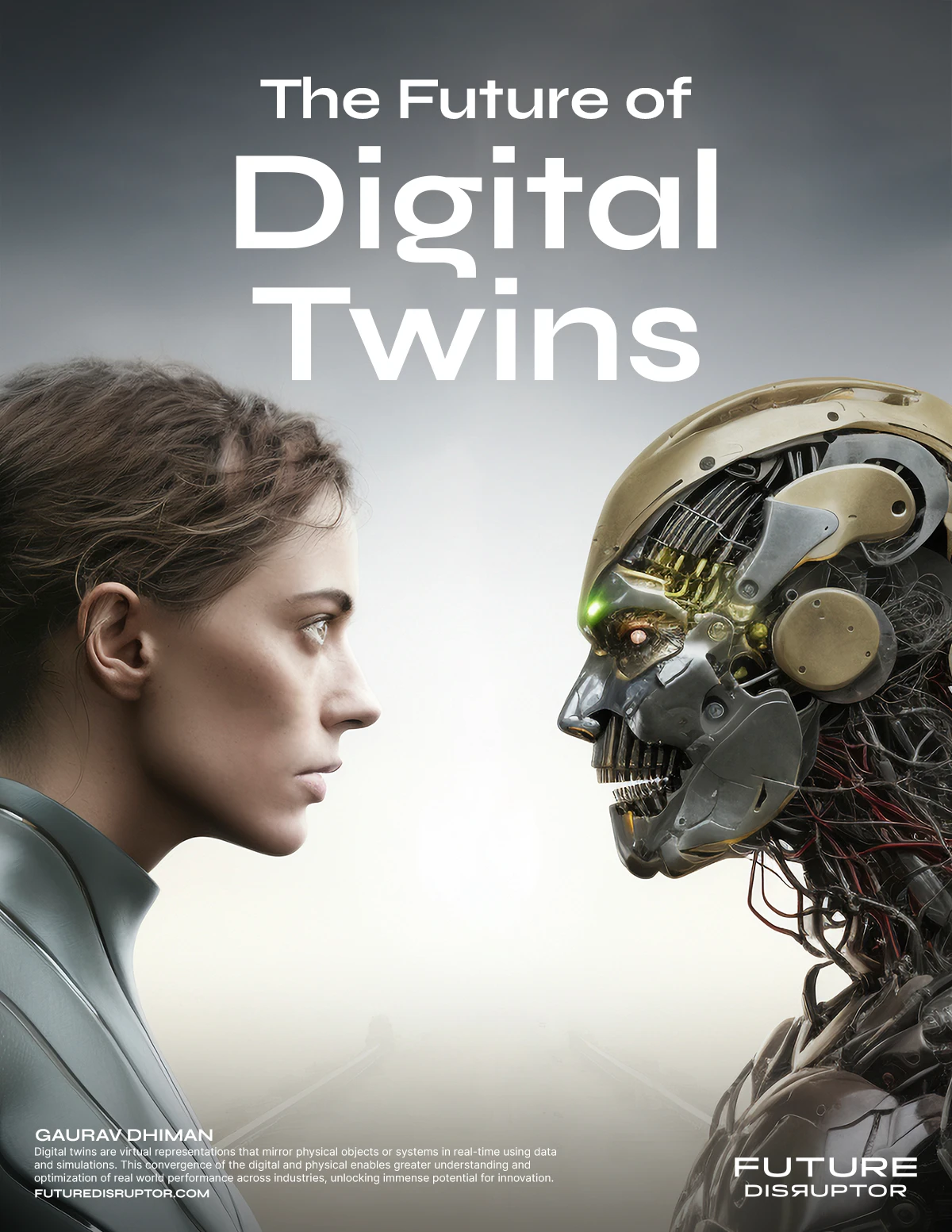
Digital twin technology creates a virtual copy of a physical object, person, or process to simulate real-world behavior and performance. It is connected to actual data sources from the environment, meaning it updates in real-time to reflect the changes in the original version. It can also use simulation, machine learning, and reasoning to help with decision-making and optimization. Examples:
- Google Maps is a digital twin of the Earth’s surface that links real-time data on traffic to help optimize your commute.
- A product twin represents a product at various life cycle stages, from initial concept design and engineering to full functionality.
- The production plant twin represents an entire manufacturing facility that can be used to monitor and improve the efficiency and quality of the production process.
- An infrastructure twin represents a physical infrastructure, such as a highway, a building, or a stadium, that can be used to study the impact of different scenarios and events on the infrastructure.
They have the potential to deliver more agile and resilient operations, and many C-suite technology executives are already exploring and investing in this technology. They can also form an enterprise metaverse, a digital and immersive environment replicating and connecting every aspect of an organization. They are also known as or related to virtual twins, cyber twins, mirror models, surrogate models, virtual prototypes, digital shadows, digital replicas, digital models, and virtual representations.
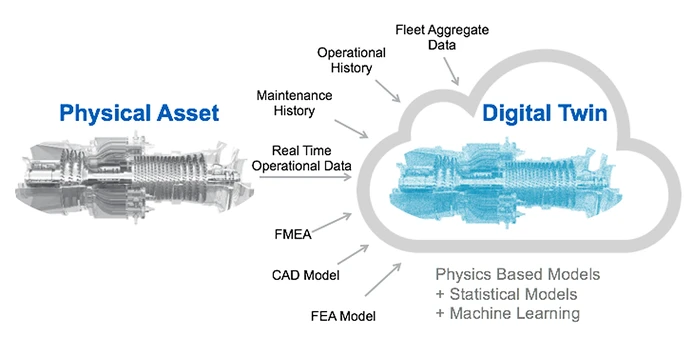
Practical Applications
Digital twins can be applied in various industries and domains to improve efficiency, performance, and innovation. Some of the practical applications are:
- Aerospace: Help engineers test and optimize the design and manufacturing of complex systems such as aircraft and spacecraft. They can also be used for simulation and training, maintenance and operations, and sales and marketing.
- Architecture: Enable architects and designers to collaborate and visualize their projects in immersive 3D environments. They can also facilitate interactive design reviews, construction planning, and building management.
- Healthcare: Help healthcare providers and pharma companies personalize patient care and treatment based on their genomes, physiology, and lifestyle. They can also help improve the operational efficiency and performance of healthcare organizations.
- Manufacturing: Enable manufacturers to monitor and optimize their products’ production process, quality, and output. They can also help with product development, prototyping, testing, and troubleshooting.
- Supply Chain: Help logistics companies plan and manage the distribution and delivery of goods and services. They can also help predict the performance and feasibility of packaging materials, enhance shipment protection, and optimize warehouse design and operations.
There are many more possibilities and opportunities for this technology to create value and impact in the future.
Importance for Our Future
Digital twin technology is important for our future because it can help us:
- Improve the design and performance of products, systems, and processes using real-time data and simulations to test and optimize various scenarios.
- Reduce costs and risks by detecting and preventing problems before they occur, avoiding expensive and time-consuming physical tests, and enhancing maintenance and operations.
- Create new opportunities and innovations using AI and machine learning to generate insights and recommendations, enabling data-driven decision-making and creativity.
- Build a more sustainable and resilient world by using digital twins to model and manage our actions’ environmental and social impacts and support the transition to low-carbon and circular economies.
It is a powerful tool that can transform how we interact with the physical world and create value and impact across various industries and domains.
How Does It Work?
The basic steps of how digital twin technology works are:
- Digitize: Create a digital model of the physical entity, including its functionality, features, and behavior. This can be done using various methods, such as 3D scanning, computer-aided design (CAD), or digital photography.
- Connect: Collect real-time data about the physical entity using sensors, such as temperature, pressure, vibration, or location. This data is then transmitted to the digital model, creating a dynamic link between the physical and digital worlds.
- Analyze: Use the digital model to understand, analyze, manipulate, or optimize the physical entity. This can be done using various techniques like simulation, machine learning, or reasoning. The analysis can help with testing, troubleshooting, forecasting, or decision-making.
- Apply: Use the insights and recommendations from the analysis to improve the performance and efficiency of the physical entity. This can be done by adjusting the parameters, settings, or actions of the physical entity or providing feedback or guidance to the users or operators.
These steps can be repeated continuously or periodically, depending on the purpose and scope of the digital twin. These systems can evolve and learn as more data and information are collected and integrated.
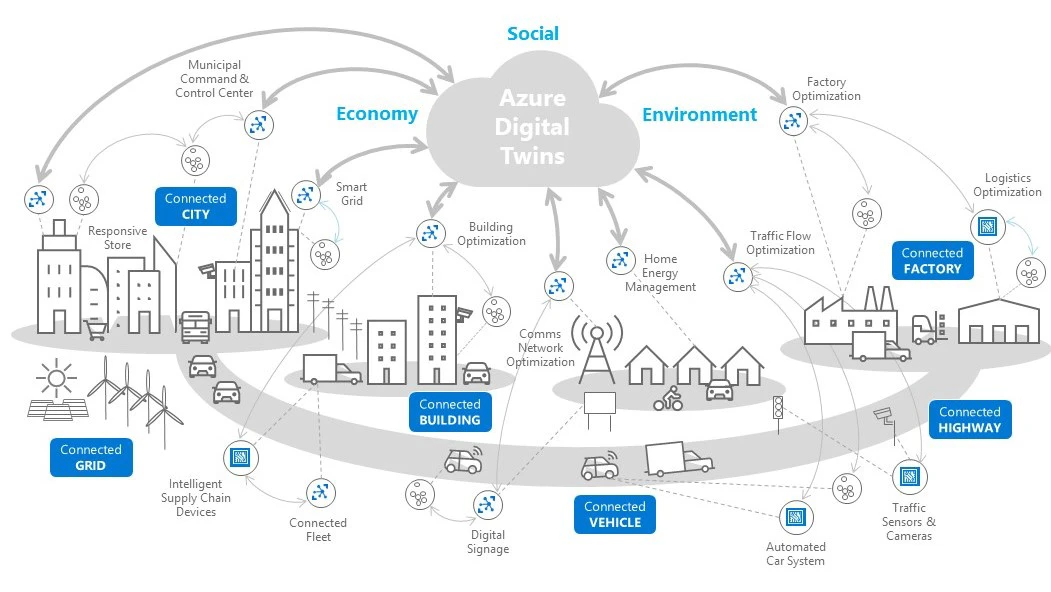
Evolve in the Future
Digital twins can evolve in the future in many ways, as it is a rapidly growing and innovative field. Some of the possible directions for the future are:
- More realistic and immersive: They can become more realistic and immersive using advanced technologies such as augmented reality (AR), virtual reality (VR), and holograms. These technologies can enable users to interact with digital twins more naturally and intuitively and experience them more immersively and engagingly.
- More intelligent and autonomous: They can become more intelligent and autonomous by using artificial intelligence (AI), machine learning (ML), and the Internet of Things (IoT). These technologies can enable digital twins to learn from data, generate insights and recommendations, and act on them without human intervention. They can also enable communication and collaboration, forming a network of interconnected digital twins.
- More scalable and accessible: They can become more scalable and accessible by using cloud computing, edge computing, and 5G networks. These technologies can enable digital twins to run on various devices and platforms and to access and process large amounts of data in real-time. Also, make them more affordable and available for multiple users and applications.
Help Organizations/Enterprises
Digital twins can help organizations and enterprises in many ways, such as:
Product Development and Manufacturing
- Optimize design and testing: Simulate product performance, identify flaws, and test different configurations before physical prototypes are built, reducing costs and development time.
- Predictive maintenance: Monitor real-time data from physical assets to anticipate failures and schedule maintenance proactively, minimizing downtime and extending equipment lifespan.
- Improve collaboration: Share and iterate on virtual models across teams, enabling faster and more efficient product development.
Supply Chain and Logistics
- Track shipments and optimize routes: Monitor goods in real-time, detect disruptions, and adapt logistics plans for improved efficiency and delivery times.
- Optimize warehouse layouts and inventory management: Simulate different configurations and inventory levels to identify the most efficient cost and resource utilization setup.
- Predict supply chain disruptions: Analyze data from various sources to anticipate potential issues like material shortages or transportation delays, allowing for proactive mitigation strategies.
Operations and Maintenance
- Improve facility energy efficiency: Analyze energy consumption patterns and identify areas for optimization, leading to cost savings and emissions reduction.
- Train and support personnel: Utilize virtual models for training simulations and assist operators with complex tasks, enhancing safety and reducing errors.
- Automate tasks and workflows: Integrate digital twins with automation systems to automate routine tasks and optimize data-driven decision-making.
Customer Service and Marketing
- Personalized customer experiences: Use data from digital twins to personalize product recommendations, predict customer needs, and offer proactive support, enhancing customer satisfaction.
- Develop targeted marketing campaigns: Analyze digital twins’ customer behavior patterns and preferences to create more effective and relevant marketing campaigns.
- Simulate product interactions and user interfaces: Test and refine product features and user interfaces in a virtual environment before real-world implementation, ensuring optimal user experience.
Driving Adoption
Factors that drive the adoption of digital twin technology in various industries and domains are:
- The advancement of emerging technologies: Technologies such as IoT, data analytics, AI, ML, cloud computing, edge computing, 5G networks, AR, VR, and holograms are enabling the creation of more realistic, intelligent, autonomous, scalable, and accessible digital twins that can provide more value and impact.
- The need for improving efficiency and performance: Improve the design and implementation of their products, systems, and processes using real-time data and simulations to test and optimize various scenarios.
- The need for reducing costs and risks: Reduce costs and risks by detecting and preventing problems before they occur, avoiding expensive and time-consuming physical tests, and enhancing maintenance and operations.
- The need for new opportunities and innovations: Create new opportunities and innovations by using AI and ML to generate insights and recommendations, enabling data-driven decision-making and creativity.
- The need for building a more sustainable and resilient world: Build a more sustainable and resilient world by using digital twins to model and manage their actions’ environmental and social impacts and to support the transition to low-carbon and circular economies.
Operational Challenges
There are many challenges in operationalizing digital twins, including:
- Data privacy and security: They generate vast amounts of data, including sensitive and confidential information about a business’s operations, customers, or products. This data must be protected from unauthorized access, misuse, or theft.
- Cost and implementation: Implementing them can be a significant investment for businesses, as it requires creating and maintaining digital models, collecting and processing real-time data, and integrating with existing systems.
- Need for skilled personnel: Creating and maintaining them requires qualified personnel with expertise and experience in various fields, such as engineering, data science, AI, and IoT.
- Integration with existing systems: They must be integrated with existing systems, such as ERP, CRM, or PLM, to ensure data consistency and compatibility. This can pose technical and organizational challenges, as different systems may have different standards, formats, or protocols.
- Ensuring extended access life cycles: They must be maintained for a long time, as they reflect the physical entities throughout their life cycles. This means they must be updated regularly and compatible with future technologies and requirements.
- Including data from many sources: They need to include data from many sources, such as sensors, cameras, GPS, or social media, to provide a comprehensive and accurate representation of the physical entities. This means that we need to deal with data quality, reliability, and availability issues and that they need to handle large and complex data sets.
- Involving the entire product value chain: The entire product value chain, from design and engineering to manufacturing and service, must create and maintain digital twins. This means that they need to facilitate collaboration and communication among different stakeholders and that they need to align with the business objectives and strategies.
- Managing design data for digital twins amongst partners and suppliers: This is a challenge when the physical product evolves, as it needs to reflect the changes and modifications made by different partners and suppliers. This means that we need to ensure data synchronization and traceability and that they need to manage data ownership and access rights.
These challenges can also be seen as opportunities for innovation and improvement, as digital twins can provide many benefits and advantages for businesses and society.
Success Stories
There are many success stories of how digital twins have been applied in various industries and domains to improve efficiency, performance, and innovation. Here are some examples:
- SoFi Stadium: Los Angeles’ 70,000-seat sport and entertainment venue is the first major stadium to use digital twin technology. It helps the stadium operators monitor and optimize the venue’s energy consumption, security, and fan experience.
- Tesla: The multinational car and clean energy company has proven its commitment to digital twins by creating a model for every car it sells. It helps Tesla track its vehicles’ performance, maintenance, and customer satisfaction and enables remote software updates and enhancements.
- Roche Tower 2: The Swiss pharmaceutical giant has used digital twins to design and construct its second tower in Basel, the tallest building in Switzerland. It helps Roche optimize the building’s structure, layout, and sustainability and facilitates collaboration and communication among the project stakeholders.
- Carl’s Jr. and Hardee’s: The fast-food chains have used digital twins to create a virtual replica of their restaurants, which can be used to test and improve the customer experience, menu, and operations. It helps the chains reduce costs, increase sales, and enhance customer loyalty.
Types
There are four main types of digital twins:
- Component twins: Digital models of individual components or parts, such as motors, sensors, switches, and valves. They can help with testing, troubleshooting, and optimizing the functionality and performance of the components.
- Asset twins: Virtual representations of a physical product rather than its parts. They can help monitor, maintain, and improve the product’s quality and reliability.
- System twins: Digital models of entire systems, such as a manufacturing line or a power plant. They can help with analyzing, managing, and optimizing the efficiency and productivity of the system.
- Process twins: Digital process models, such as manufacturing or supply chains. They can help with simulating, forecasting, and enhancing the outcomes and performance of the process.
Advantages
Here are some of the advantages of digital twin technology:
- Real-time data and predictive analytics: They gather data from sensors embedded in the physical system, providing a real-time view of its performance. AI algorithms then analyze this data to predict potential issues and optimize operations. Imagine a wind turbine’s digital twin expecting wear and tear on its blades, enabling preventive maintenance and maximizing energy generation.
- Reduced downtime and increased efficiency: They help identify potential problems before they occur, which can help prevent costly downtime and optimize resource utilization. For example, a factory’s digital twin might pinpoint inefficiencies in production lines, allowing for adjustments that boost overall output.
- Virtual testing and simulations: They act as virtual testbeds, allowing engineers and decision-makers to experiment with different scenarios and configurations without impacting the actual system. This lets them optimize product designs, test control strategies, and predict the outcome of critical decisions before implementation.
- Improved collaboration and communication: They provide a shared platform for stakeholders across disciplines to visualize and analyze data, fostering better collaboration and communication. Imagine architects, engineers, and construction workers all working with a digital twin of a building, ensuring seamless coordination and smoother project execution.
- Faster product development cycles: They can dramatically shorten product development cycles by enabling virtual prototyping and testing. This allows companies to bring innovative products to market faster and adapt to changing demands with greater agility.
- Improved product quality and reliability: Identifying potential design flaws and manufacturing defects early on can help enhance the quality and reliability of the product. This translates to fewer product recalls, reduced warranty costs, and enhanced customer satisfaction.
- Reduced energy consumption: They can optimize energy consumption in buildings, factories, and other systems. This can lead to significant cost savings and reduced environmental impact. For instance, a building’s digital twin might suggest heating and cooling systems adjustments, minimizing energy waste.
- Improved resource management: They can help optimize resource utilization, such as water, materials, and fuel, by identifying areas of waste and inefficiency. This promotes sustainability and reduces the environmental footprint of operations.
Disadvantages
Here are some of the disadvantages of digital twin technology:
- Cost and complexity: Implementing and maintaining a sophisticated digital twin can be expensive. It requires investment in sensors, data infrastructure, modeling software, and skilled personnel to manage and interpret the data. The complexity of creating and maintaining an accurate digital twin increases with the size and intricacy of the physical system being modeled.
- Data security and privacy risks: They rely on collecting and transmitting vast amounts of data, often in real-time. This raises concerns about data security and privacy, as cyberattacks or unauthorized access could compromise sensitive information. Ensuring proper data governance and implementing robust cybersecurity measures is crucial to mitigate these risks.
- Limited interoperability: Different digital twin platforms and software often use proprietary formats and standards, making it challenging to share data and integrate with other systems. Lack of interoperability can limit the potential benefits of digital twins, as it hinders collaboration and information exchange.
- Dependency on data quality: Its accuracy and effectiveness heavily depend on the quality of the data it receives. Inaccurate or incomplete data can lead to misleading simulations and unreliable predictions. Investing in robust data collection and validation processes is essential to ensure trustworthiness.
- Maintenance and upkeep: They are not static models; they must be constantly updated to reflect changes in the physical system they represent. This requires ongoing care and effort to ensure they remain accurate and relevant. Neglecting to update them can render them useless and negate their potential benefits.
- Potential for misuse: The powerful capabilities of digital twins raise concerns about potential abuse, such as surveillance or manipulation of physical systems. Ethical considerations and responsible development practices are crucial to ensuring they are used for good.
Ethical Concerns
Digital twins raise some ethical concerns, such as:
Privacy and Data Security
- Surveillance and control: Who owns and controls this data? Could it be used for intrusive monitoring or manipulation of individuals?
- Data breaches and misuse: How vulnerable is this data to cyberattacks, and what safeguards are in place to prevent abuse?
- Algorithmic bias: Can the algorithms used to analyze data perpetuate existing biases, leading to unfair or discriminatory outcomes?
Transparency and Explainability
- Black box problem: How can we understand and trust the decisions made by the digital twin, especially when they have significant real-world consequences?
- Accountability: Who is responsible for errors or biases generated by the digital twin?
Environmental Impact
- Energy consumption: Running complex simulations and powering data centers can be energy-intensive.
- E-waste: The rapid development of technology can lead to increased electronic waste.
Societal Impact
- Exacerbate inequality: Access to the benefits of digital twins may not be equally distributed, potentially widening the gap between those who have and those who have not.
- Dehumanization: Overreliance on digital twins for decision-making could lead to a disconnect from the human element and a diminished appreciation for lived experiences.
Governance and Regulation
To govern and regulate digital twins, we must establish clear and consistent rules and standards to address these challenges and ensure this technology’s responsible and beneficial use. Some of the possible ways to do this are:
- Developing international and national frameworks: To provide guidance and best practices for developing and implementing digital twins. These frameworks should be based on transparency, accountability, fairness, and respect. They should involve the participation and consultation of various stakeholders, such as governments, businesses, academia, civil society, and users.
- Adopting and harmonizing technology standards: To ensure digital twins’ interoperability, compatibility, and quality. These standards should cover data collection, processing, sharing digital model creation and maintenance, and integration and operation.
- Enforcing and monitoring compliance: Use rules and standards for digital twins and provide mechanisms for resolving disputes and addressing grievances. This can be done by establishing independent and impartial authorities, agencies, or bodies that can oversee and audit the use and by providing legal and ethical frameworks for liability and accountability.
History
The history of digital twin technology can be traced back to the following milestones:
- 1960s: NASA used basic twinning ideas for space programming, such as creating physically duplicated systems at ground level to match the systems in space. An example is the Apollo 13 mission, where NASA used a digital twin to assess and simulate conditions on board the spacecraft.
- 1991: David Gelernter published Mirror Worlds, a book that anticipated the concept of digital twins.
- 1998: The phrase ‘digital twin’ was first mentioned in a paper by Alan Alda and his colleagues, who created a digital copy of Alda’s voice.
- 2002: Michael Grieves introduced the digital twin as the conceptual model underlying product lifecycle management (PLM) at a conference in Michigan.
- 2010: John Vickers of NASA popularized the term ‘digital twin’ in a Roadmap Report and applied the concept to improve the physical-model simulation of spacecraft.
- 2017: Digital twins became one of the top strategic technology trends, as the Internet of Things (IoT) enabled digital twins to become cost-effective and widely used in various industries and domains.
Tools and Services
Many software applications and platforms enable users to create, manage, and use digital twins of physical objects, persons, or processes that include:
- Microsoft Azure Digital Twins: This is a cloud-based service that allows users to create comprehensive digital models of entire environments, such as buildings, factories, farms, or cities. Users can connect inputs from IoT devices or business systems to digital models and use query APIs and data analytics to gain insights and recommendations.
- Autodesk Digital twin: This is a software solution that helps users design and construct digital twins of physical assets, such as products, facilities, or infrastructure. Users can use computer-aided design (CAD) tools, data sources, and simulation tools to create and visualize digital twins and use them for collaboration, optimization, and innovation.
- XMPro: This application development platform enables users to build sophisticated applications that combine event detection, advanced real-time analytics, and prescriptive actions. Users can use XMPro to create and use digital twins of industrial assets, such as machines, equipment, or processes.
- SAP Leonardo Internet of Things: This cloud-based platform helps users integrate IoT data into their core business processes. Users can use SAP Leonardo to create and use digital twins of their products, assets, or fleets and use them to improve productivity, quality, and customer satisfaction.
How to Get Started?
To start to develop and deploy digital twin technology, you need to:
- Define your objectives and outcomes: Identify the business problems or opportunities you want to address with digital twins and the expected benefits and value you want to achieve.
- Select your use cases and scope: Select the specific use cases and scenarios relevant and feasible for your objectives and outcomes and define the scope and boundaries of your model.
- Choose your partners and providers: Choose the partners and providers that can help you with the development and implementation of your digital twins, such as software vendors, data providers, or consultants.
- Create your digital models and data sources: Create the digital models of your physical entities using various methods such as 3D scanning, CAD, or digital photography. You must also collect and connect the data sources that will feed your digital twins, such as sensors, cameras, GPS, or social media.
- Build and test your digital twins: Build and test your digital twins using various tools and platforms that enable creation, management, and use. You must also validate and verify the accuracy and reliability and measure their performance and impact.
- Deploy and scale your digital twins: Deploy and scale your digital twins by integrating them with your existing systems and processes and expanding their capabilities and functionalities. You must also maintain and update to ensure their security and privacy.
Related Terms
Some of the terms related to digital twins are:
- Digital model: A digital representation of the functionality, features, and behavior of a physical entity, such as a product, system, or process.
- Data source: A source of data that provides information about the physical entity, such as sensors, cameras, GPS, or social media.
- Simulation: A technique that uses the digital model and the data source to mimic the behavior and performance of the physical entity under various conditions and scenarios.
- Machine learning: A branch of artificial intelligence that uses data and algorithms to learn from patterns and make predictions or recommendations.
- Reasoning: A technique that uses logic and rules to draw conclusions and make decisions based on the data and the simulation.
- Insight: A piece of information or knowledge that is derived from the data, the simulation, the machine learning, or the reasoning and that can help improve the physical entity.
- Component twin: A digital model of an individual component or part of a system or product, such as a motor, sensor, switch, or valve.
- Asset twin: A digital model of a physical product rather than its parts, such as a car, a phone, or a machine.
- System twin: A digital model of an entire system, such as a manufacturing line, a power plant, or a network.
- Process twin: A digital process model, such as a manufacturing process, a supply chain, or a business process.
- Enterprise metaverse: A digital and often immersive environment replicating and connecting every aspect of an organization, such as products, systems, processes, and people.
Learn more
Research papers
- Digital Twin: Enabling Technologies, Challenges and Open Research: Provides an overview of the concept, definition, history, applications, challenges, and future directions of digital twin technology. It also reviews and categorizes publications relating to digital twins in various research areas, such as manufacturing, healthcare, and smart cities.
- A comprehensive review of digital twin — part 1: modeling and twinning enabling technologies: Analyzes the roles of different modeling techniques and twinning-enabling technologies in digital twins. It also classifies them into two main categories: physical-to-virtual and virtual-to-physical, based on the direction of the data flow.
- Systematic review of digital twin technology and applications: Summarizes the concept and definition of digital twin, as utilized by scholars and researchers in various fields of industry. It also explains the internal association between digital twins and related technologies and identifies the history of the four stages of digital twin development.
Books
- Digital Twin Technologies and Smart Cities: Covers the fundamentals and applications of digital twins in smart cities. It also addresses the challenges and solutions for its development and deployment in urban environments.
- Digital twin Development and Deployment on the Cloud: A comprehensive guide for developing and deploying digital twins on the cloud. It also covers its architecture, data management, security, and analytics.
- Digital twin Driven Smart Manufacturing: Focuses on the role of digital twin technology in intelligent manufacturing. It also presents the methods and models for creating and using them for product design, production planning, process control, and quality assurance.
Articles
- How Will Digital Twins Evolve in the Future?: Explores the future trends and directions of digital twins, such as more realistic and immersive, more intelligent and autonomous, and more scalable and accessible. It also highlights some of the challenges and opportunities.
- Top 8 Digital twin Benefits: Lists some of the main advantages of digital twins, such as improved design and performance, reduced costs and risks, new opportunities and innovations, and a sustainable and resilient world. It also provides some tips and best practices for getting started.
I hope you enjoyed this insightful discourse. I believe that open dialogue and diverse perspectives are essential for fostering a deeper understanding of complex issues. Therefore, encourage you to share your thoughts and opinions in the comments below. Engage with other readers, challenge assumptions, and contribute to a rich and meaningful discussion. Your insights are valuable, and welcome you to participate actively in this intellectual exchange.
Together, we can explore new ideas, expand our horizons, and generate fresh perspectives.
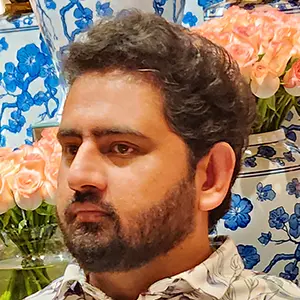
Learner-in-Chief at Future Disruptor. A futurist, entrepreneur, and management consultant, who is passionate about learning, researching, experimenting, and building solutions through ideas and technologies that will shape our future.
Subscribe to the Future Disruptor newsletter.
Leave a Reply